A significant limitation of standard Model Predictive Control applications is their sensitivity to changes in the process they are designed to control. It is typical for the behaviour of a process to change overtime, thus, if left unchanged, the accuracy of the underlying MPC model in an MPC application gradually declines and so the controller effectiveness drops.
To circumvent this limitation Spiro MPC incorporates an effective method for automatically updating the MPC model on a continuous basis in response to changes in process conditions. The result is that Spiro MPC delivers sustained benefits without a need for significant maintenance. This is achieved through the following:
- Automated process step testing while maintaining process within specifications at all times and not compromising optimisation objective
- Real-time recursive model identification
- Seamless integration between controller and model identification; building/updating models is performed online, as is the functionality for pushing new models to the controller
- Masking is applied to retain existing high confidence models
- Constraints are applied to the model identification to capture prior process knowledge
PART 1: DATA GENERATION/INTEGRATED CONSTRAINED CLOSED LOOP TESTING
Without disturbing normal operation, Spiro MPC stimulates the process to generate statistically rich data needed to build high confidence models.
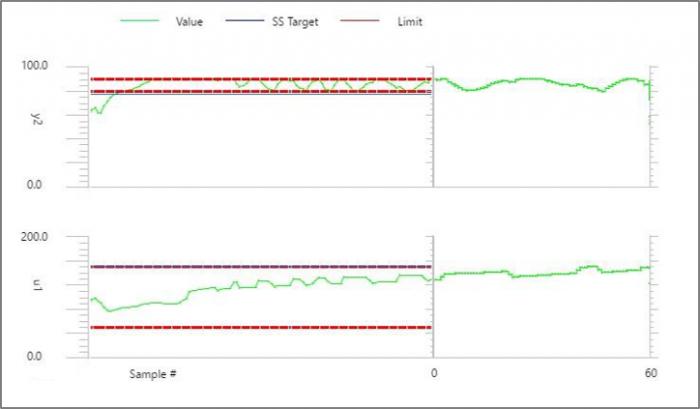
The above screen shot shows step testing in action. With Spiro MPC step testing is fully automated and adheres to all defined constraints while still driving the process to the targets during testing. Continuous monitoring is therefore not required. The figure shows the steps being made in u1 while keeping y1 within the constraints (red lines).
Following traditional methods for step testing, the concern is that product quality and energy consumption will be worse as the process may drift away from the targets. However, with Spiro MPC, the process operates close to targets even when stepping and so any ‘cost’ to performing a step test is minimal. A further benefit of the methodology is that it dramatically reduces the complexity of updating the MPC model; no longer is this exclusively the task of an experienced APC engineer.
PART 2: ONLINE MODEL IDENTIFICATION
The data generated by step testing is processed in real time with recursive identification algorithms that incrementally build a robust model. This means that with Spiro MPC the entire process of building new models or updating existing ones is performed online. The configuration and visualization of the evolving models is fully integrated in the standard MPC visualization, as is the functionality that enables the user to push new models to the controller. This is a dramatically more efficient process than the traditional method which typically involves gathering data in collection modules, transporting the data offline to build models, to then transport back updated models.
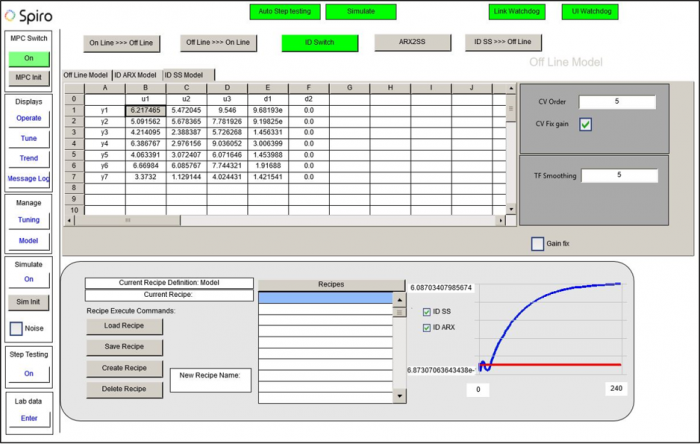
Figure 2: Spiro MPC model manager interface
PART 3: CAPTURING PRIOR PROCESS KNOWLEDGE
To ensure best performance, users can impose constraints on the model identification. Using a simple interface, users can enter prior information such as process delays and gains which will then be incorporated in the identification of the model.
CONCLUSION
This unique adaptive model functionality is yet another way for us to deliver a highly flexible MPC solution. With this latest Spiro MPC technology, implementation and maintenance of MPC is no longer a complex and expensive project.
What’s more, in addition to adaptive model functionality, Spiro MPC includes an integrated performance monitoring tool which assists in the identification of any degradation in performance. Together these tools maintain performance benefits while reducing any maintenance burden.
You can request a personalised demo of Spiro MPC or find more information here.